In today’s fast-paced digital era, ensuring software quality is not just a priority but a necessity for businesses aiming to stay competitive. Traditional Quality Assurance (QA) methods, while effective, often face challenges in keeping up with the rapid pace of software development and the ever-increasing complexity of modern applications. However, with the advent of Artificial Intelligence (AI), QA transformation has reached a new frontier. In this blog, we’ll explore how AI-driven QA transformation is revolutionizing the way organizations approach software testing, delivering higher efficiency, accuracy, and agility.
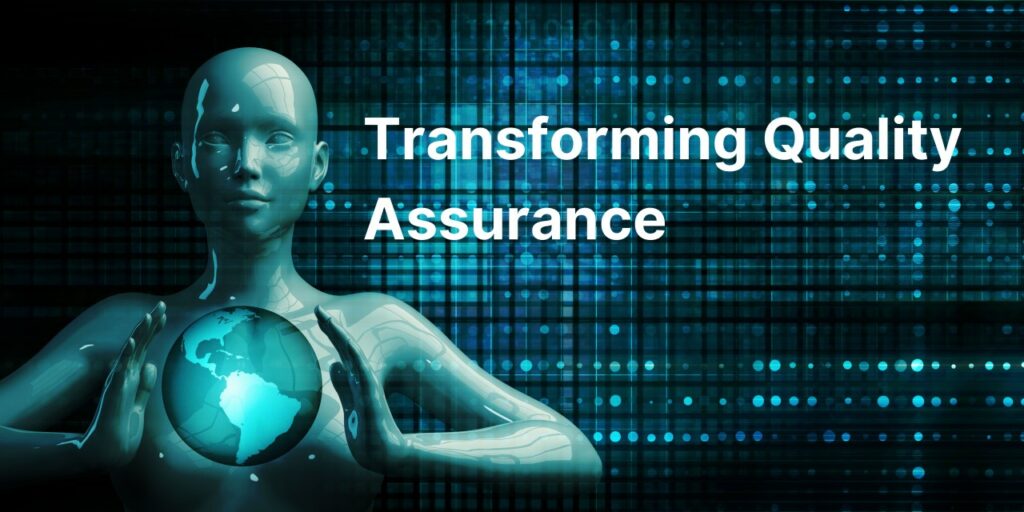
Understanding AI in QA:
To grasp the significance of AI-driven QA transformation, it’s essential to understand the role of AI in the QA process. AI in QA refers to the integration of artificial intelligence technologies and techniques to enhance various aspects of quality assurance. These technologies include machine learning algorithms, natural language processing, predictive analytics, and more. By leveraging AI, organizations can automate repetitive tasks, uncover hidden patterns in testing data, and predict potential defects with unprecedented accuracy.
Key Components of AI-Driven QA Transformation:
- Data Collection and Preprocessing: Gathering diverse testing data from various sources, including user feedback, logs, and performance metrics. Preprocessing data to ensure quality and relevance for AI analysis, including data cleaning, normalization, and feature engineering.
- Test Case Prioritization and Optimization: Utilizing AI algorithms to prioritize test cases based on factors such as risk, complexity, and business impact. Optimizing testing efforts by focusing on high-priority test cases and minimizing redundant testing.
- Automated Defect Detection and Analysis: Implementing AI-powered tools to detect, classify, and analyze defects in software applications. Accelerating the debugging process by automatically identifying and prioritizing critical defects, reducing manual intervention.
- Predictive Analytics for QA Improvement: Leveraging AI models to analyze historical testing data and forecast future QA trends. Enabling proactive quality improvement strategies, such as predicting potential defects before they occur and optimizing testing resources.
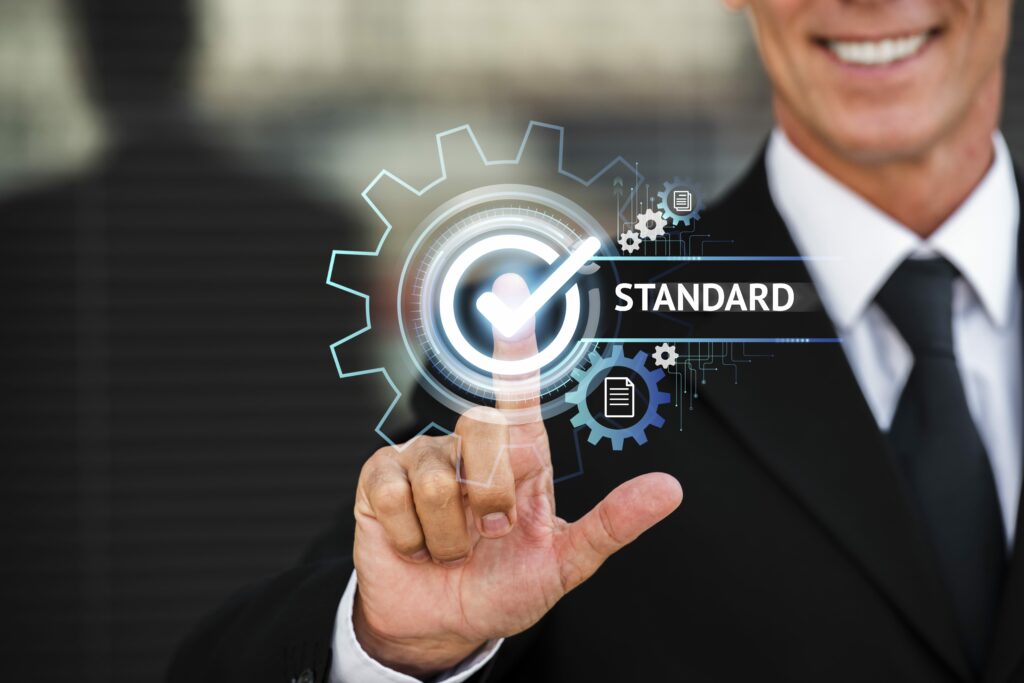
Implementing AI in QA: While the benefits of AI-driven QA transformation are compelling, successful implementation requires careful planning and execution. Organizations must assess their readiness for AI adoption, choose appropriate tools and technologies, integrate AI seamlessly into existing processes, and provide adequate training and support to their teams. It’s crucial to emphasize that AI is not a replacement for human testers but rather a complement, augmenting their capabilities and enabling them to focus on higher-value tasks.
Best Practices for AI-Driven QA Transformation: To maximize the effectiveness of AI-driven QA transformation initiatives, organizations should follow best practices, including:
- Defining clear objectives and Key Performance Indicators (KPIs) to measure the effectiveness of AI initiatives.
- Continuously monitoring and refining AI models and processes to adapt to evolving testing requirements and challenges.
- Fostering collaboration and communication between QA and development teams to ensure alignment and synergy in AI adoption efforts.
- Ensuring compliance with data security and privacy regulations to protect sensitive testing data and maintain trust with stakeholders.
AI-driven Quality Assurance (QA) Transformation, various types of AI technologies and methodologies are utilized to enhance the QA process. These types can be categorized based on their specific functions and applications within QA transformation:
- Machine Learning-based Testing:
- Regression Testing: Machine learning algorithms are employed to automate the execution of regression test suites, ensuring that changes in code do not adversely impact existing functionality.
- Predictive Maintenance Testing: Machine learning models are utilized to predict potential defects or areas of weakness in the software, allowing QA teams to proactively address them before they escalate into major issues.
- Natural Language Processing (NLP) for Test Automation:
- Automated Test Case Generation: NLP techniques are utilized to analyze requirements documents and automatically generate test cases, reducing manual effort and improving test coverage.
- Chatbot Testing: NLP-based chatbots are employed to simulate user interactions with the software, allowing QA teams to test the system’s response to various user inputs and scenarios.
- Computer Vision for UI Testing:
- Visual Regression Testing: Computer vision algorithms are used to compare screenshots of the UI before and after code changes, detecting any visual discrepancies or regressions.
- Accessibility Testing: Computer vision is leveraged to analyze UI elements and ensure compliance with accessibility standards, such as proper labeling of images and text.
- Predictive Analytics for Test Case Prioritization:
- Risk-based Testing: Predictive analytics models are employed to prioritize test cases based on their potential impact on critical business functions or areas of the application most prone to defects.
- Resource Optimization: Predictive analytics is used to forecast resource requirements for testing activities, optimizing allocation of QA resources and minimizing bottlenecks.
- Robotic Process Automation (RPA) for Test Execution:
- Automated Test Execution: RPA tools are utilized to automate repetitive testing tasks, such as data entry and test case execution, freeing up QA teams to focus on more strategic testing activities.
- Cross-platform Testing: RPA bots are employed to execute tests across multiple platforms and environments, ensuring consistency and compatibility of the software across different devices and configurations.
Case Studies: Successful AI-Driven QA Transformations: Real-world case studies provide valuable insights into the tangible benefits and challenges of AI-driven QA transformation. By examining successful implementations across diverse industries, organizations can glean practical lessons and inspiration for their own QA journey. Case studies highlight the transformative impact of AI on QA processes, including increased efficiency, improved accuracy, and enhanced customer satisfaction.
Future Trends in AI-Driven QA: As technology continues to evolve, the future of AI-driven QA holds exciting possibilities. Emerging trends include advancements in AI capabilities, integration with DevOps practices, and the evolving role of QA professionals in an AI-powered testing landscape. Organizations that embrace these trends and invest in AI-driven QA transformation will be better equipped to meet the demands of the digital age and deliver high-quality software products at scale.
Conclusion: In conclusion, AI-driven QA transformation represents a paradigm shift in the way organizations approach software quality assurance. By harnessing the power of AI, organizations can unlock new levels of efficiency, accuracy, and innovation in their QA processes. With experts leading the way and best practices guiding implementation, the future of QA is brighter than ever. As we continue to embrace AI-driven QA transformation, we can look forward to a future where software quality is not just a goal but a reality.
Those are yours alright! . We at least need to get these people stealing images to start blogging! They probably just did a image search and grabbed them. They look good though!