The field of data analytics is evolving rapidly, driven by technological advancements and the increasing volume and complexity of data. As organizations seek to gain a competitive edge, they are turning to data analytics to uncover valuable insights and make informed decisions. In this blog post, we’ll explore the top 7 data analytics trends that are shaping the future, providing a more in-depth analysis of each trend.
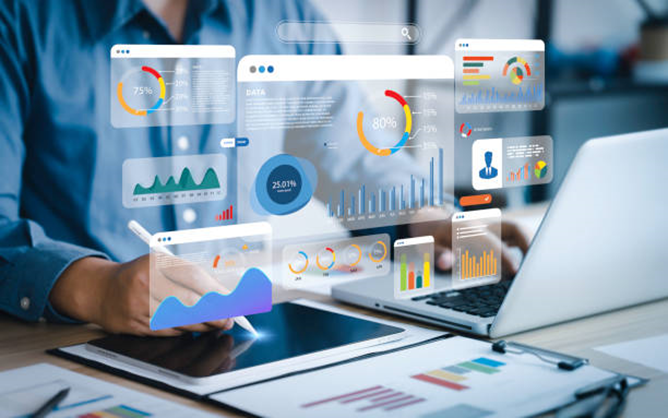
Artificial Intelligence and Machine Learning (AI/ML)
AI and ML are becoming increasingly integrated into data analytics workflows. These technologies enable machines to learn from data, identify patterns, and make predictions without explicit programming. This has significant implications for tasks such as:
Predictive analytics: Forecasting future trends and outcomes. For example, retailers can use AI/ML to predict customer demand and optimize inventory levels.
Natural language processing: Analyzing and understanding human language. This is essential for tasks such as sentiment analysis, ai chatbots, and customer service.
Computer vision: Processing and understanding visual information. Applications include image recognition, object detection, and facial recognition.
AI/ML algorithms are becoming more sophisticated and capable of handling larger and more complex datasets. This has led to the development of new techniques such as deep learning, which is particularly effective for tasks involving unstructured data like images and text.
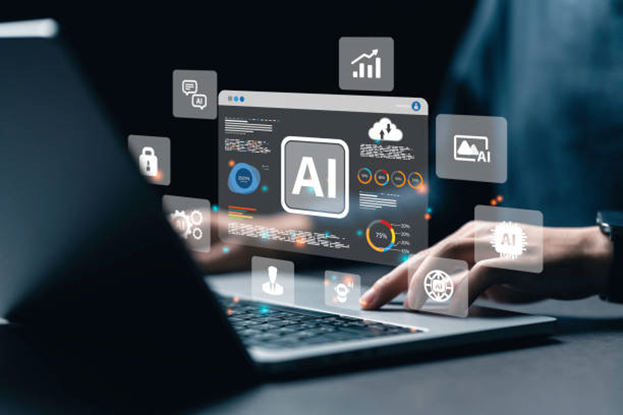
Data Democratization
Data democratization refers to the practice of making data accessible to a wider range of people within an organization. By breaking down silos and providing tools that empower users to explore and analyze data, organizations can foster a data-driven culture and encourage innovation.
One of the key challenges in data democratization is ensuring that data is accessible to users with varying levels of technical expertise. This requires providing intuitive and easy-to-use tools, as well as providing training and support. Additionally, organizations must address concerns related to data privacy and security to ensure that sensitive data is protected.
Cloud-Based Analytics
The adoption of cloud-based analytics platforms is on the rise, offering scalability, flexibility, and reduced infrastructure costs. Cloud providers offer a wide range of data analytics tools and services, making it easier for organizations to access and analyze data.
Cloud-based analytics platforms are particularly well-suited for organizations that need to process large volumes of data or that have rapidly changing data requirements. By leveraging the scalability and flexibility of the cloud, organizations can avoid the upfront costs of purchasing and maintaining their hardware and software.
Internet of Things (IoT) Analytics
As the number of connected devices continues to grow, IoT analytics is becoming increasingly important. By analyzing data generated by IoT devices, organizations can gain valuable insights into operations, customer behavior, and market trends.
IoT analytics can be used to optimize operations, improve customer experiences, and develop new products and services. For example, manufacturing companies can use IoT data to monitor equipment performance and identify potential maintenance issues. Retailers can use IoT data to track inventory levels and optimize store layouts.
Edge Computing
Edge computing involves processing data closer to its source, rather than relying on a centralized cloud infrastructure. This can reduce latency and improve performance for applications that require real-time data processing.
Edge computing is particularly well-suited for applications that require low latency, such as autonomous vehicles, industrial automation, and smart grid systems. By processing data at the edge, organizations can reduce the amount of data that needs to be transmitted to the cloud, which can improve performance and reduce costs.
Augmented Analytics
Augmented analytics combines AI and automation to enhance the data analytics process. This includes features such as natural language processing for querying data, automated data preparation, and AI-driven insights.
Augmented analytics can help organizations get more value from their data by automating routine tasks and providing insights that would be difficult or impossible to obtain using traditional methods. For example, augmented analytics can be used to identify patterns in data that are too complex for humans to detect, or to generate automated reports that can be used to monitor performance and identify trends.
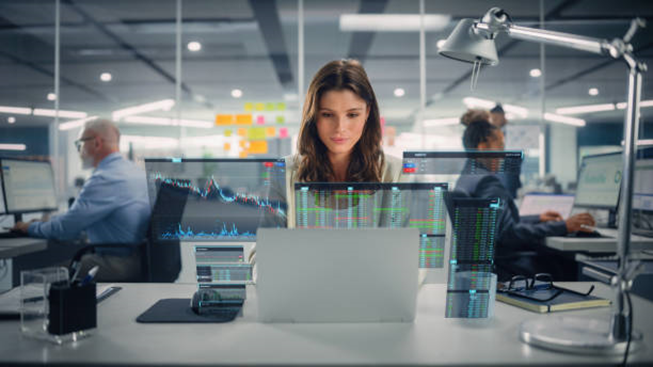
Data Ethics and Privacy
As organizations collect and analyze increasing amounts of data, data ethics, and privacy are becoming major concerns. Ensuring responsible and ethical use of data is essential for building trust with customers and stakeholders.
Data ethics and privacy are complex issues that require careful consideration. Organizations must develop policies and procedures to ensure that data is collected, used, and shared responsibly and ethically. Additionally, organizations must comply with relevant laws and regulations, such as the General Data Protection Regulation (GDPR) and the California Consumer Privacy Act (CCPA).
Conclusion
The field of data analytics is rapidly evolving, driven by technological advancements and the growing importance of data-driven decision-making. By embracing these trends, organizations can unlock the full potential of their data and gain a competitive advantage.